Unlocking Durable Value in Enterprise-Grade AI
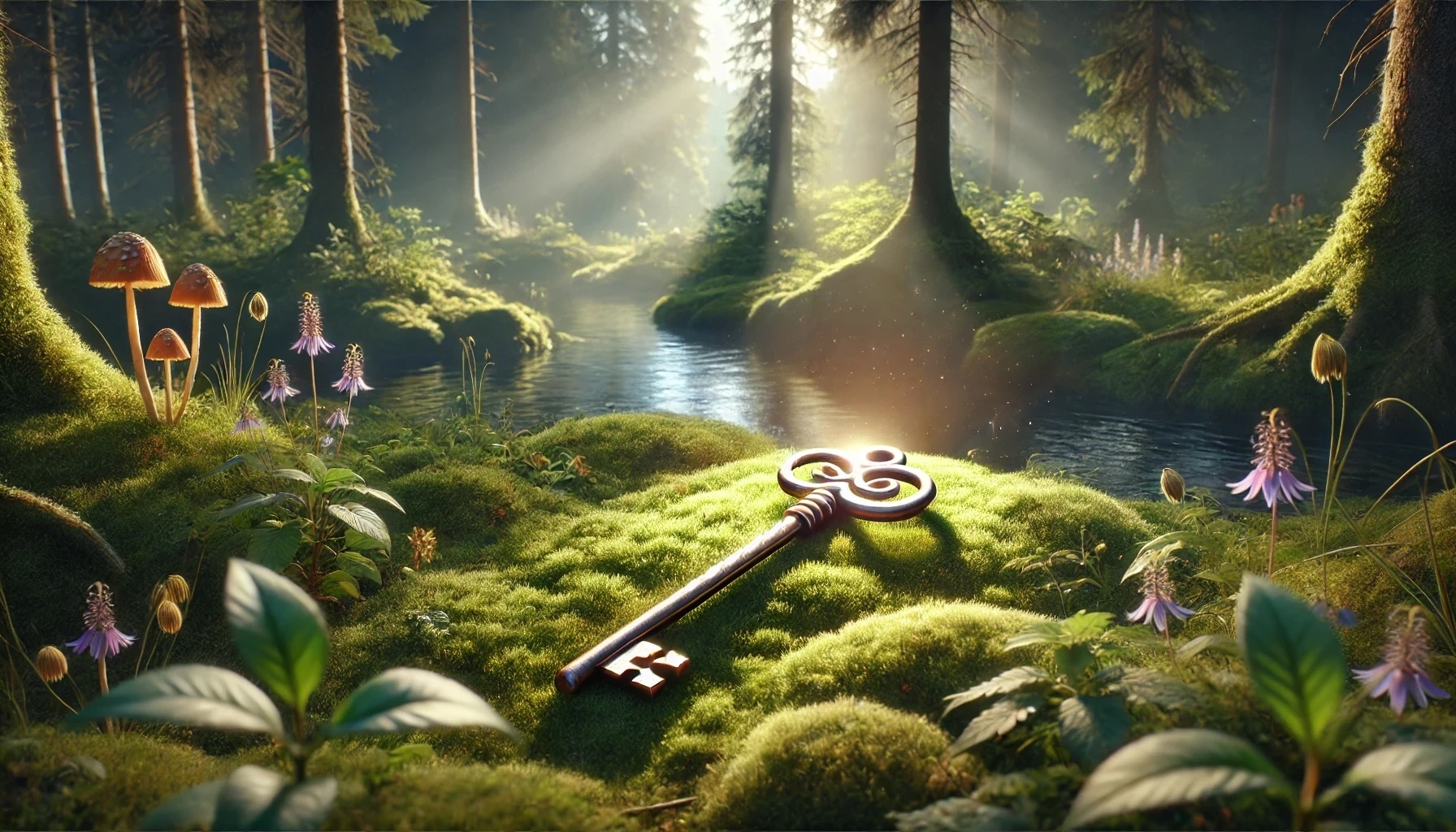
Artificial intelligence (AI) is at the forefront of innovation, transforming industries by enabling automation, enhancing decision-making, and delivering personalized experiences. However, the true success of AI within enterprise environments depends not just on the sophistication of the models themselves, but how well AI is built to integrate and scale within an organization’s existing systems.
Despite the buzz around AI, we are still in the early stages of widespread enterprise adoption. AI is being used in specific cases like chatbots and marketing tools, but the transformational potential of AI has not yet had an impact on large-scale operations.
AI adoption at the enterprise level is far from straightforward. It’s not about deploying off-the-shelf tools but navigating the unique challenges of scale, integration, privacy, and business alignment to ensure that AI solutions provide sustainable, long-term value.
How Does This Differ From General Enterprise B2B Adoption?
While enterprise adoption of B2B SaaS solutions and AI tools shares similarities—such as integrating new technologies into existing workflows and managing organizational change—there are a few key differences:
- Customization and Data Dependency: AI solutions must be tailored to specific workflows, requiring model training on proprietary data and ongoing model fine-tuning. SaaS tools, in contrast, often function as plug-and-play solutions.
- Continuous Learning and Adaptation: AI systems thrive on dynamic data inputs to improve predictions and decision-making over time, unlike SaaS tools, which typically remain static after deployment.
- Cross-Functional Collaboration: Implementing AI often demands coordination across departments (e.g., operations, IT, data science) to effectively embed insights into workflows, this practice is less common in SaaS onboarding.
- Regulatory and Compliance Considerations: Industries like healthcare and finance add another layer of complexity to AI integration due to strict legal frameworks, which require explainability and auditability for AI decisions.
- Strategic Importance: AI systems fundamentally reshape decision-making processes which has a more direct impact on core business operations compared to SaaS tools, which usually support existing workflows.
These differences elevate AI from a tool to a critical component of organizational strategy, requiring more investment in both human and technological resources.
A Holistic Approach to AI Adoption
To understand this better, let’s look at what happens when there’s minimal interaction at the enterprise level—what we might call "light-touch AI solutions." Light-touch AI solutions are software applications that require minimal touch within an organization's existing systems. Typically featuring user-friendly interfaces and built atop existing AI models, these products offer a quick and easy way for enterprises to adopt new technologies without extensive infrastructure changes or security parameters.
At the team level, these solutions can be highly beneficial. They enable groups to experiment with AI capabilities without the need for deep technical expertise or major changes to existing systems. Teams can quickly automate routine tasks, enhance productivity, and foster innovation within their specific domains. The accessibility of these tools lowers the barriers to entry, allowing team members to focus on their core responsibilities while leveraging AI to augment their work. This agility can lead to short-term gains and is particularly useful for pilot projects or in areas where rapid iteration is valued.
However, in enterprise environments, AI adoption is not a plug-and-play solution. It requires a more holistic approach, which ensures AI becomes a fully embedded part of the organization’s processes.Thoughtful companies building these solutions go deeper. They work on integrating AI models with legacy systems, regulatory environments, and workflow-specific needs of large enterprises. Essentially, they ensure that AI applications are not just standalone tools but are intricately woven into the fabric of an organization’s processes.
This approach matters because they tap into deep revenue streams and create long-term value. Enterprises don’t just care about functionality; they care about solving their unique problems.
AI models are becoming commoditized, but integrating models into real workflows of large organizations is where value is created and captured. When you solve for those complexities – whether it’s in healthcare, finance, or customer support–enterprises are willing to invest significantly because the solution becomes embedded in their day-to-day operations.
Examples in Practice
Traditional AI Limitations in Manufacturing Supply Chains:
Traditional AI models often fail to adapt to the complex workflows and dynamic decision-making required in modern supply chains, particularly lacking in cross-functional collaboration and data flow integration with systems like ERP.
An interesting company we found while researching Supply Chain AI is Lyric, backed by Primary Ventures.
Lyric's platform is designed to help enterprises make AI a core part of their supply chain decision-making processes. Unlike many other AI tools that simply provide models or off-the-shelf solutions, Lyric integrates AI deeply into the workflows of organizations, enabling them to move from reactive to proactive supply chain management:
- Collaborative Environment for AI-Driven Solutions: It creates a collaborative space where data scientists, operations researchers, and supply chain engineers jointly build AI-driven decision products, tailored to address specific supply chain challenges such as inventory optimization and demand forecasting.
- Seamless Integration with Legacy Systems: Lyric offers pre-built, customizable tools that integrate seamlessly with legacy systems like ERP and TMS platforms, embedding AI recommendations directly into daily operations without requiring extensive data science expertise.
- Automated AI Model Development and Maintenance: Through its ML Workspace, Lyric automates the complex tasks of building and maintaining AI models, allowing teams to generate reliable predictive models that adapt to evolving conditions, ultimately enhancing efficiency and resilience in supply chain decision-making.
Altana’s AI: Transforming Global Supply Chain Visibility
One of the more advanced examples of enterprise AI integration is Altana which has raised $200M from the likes of March Capital & GV Ventures. The company applies AI to global supply chains, enabling enterprises, governments, and logistics providers to enhance security, sustainability, and resilience. Founded by a team of experts from global trade and government sectors, Altana offers a unique approach to supply chain management by using AI to address the challenges of globalization and its effects on logistics networks.
Altana’s platform is powered by a hub-and-spoke AI system that connects and learns from logistics and business-to-business data. This system offers insights from data without compromising its confidentiality—providing organizations with a shared view of global supply chain networks while keeping sensitive data secure.
For example, Altana’s AI models can:
- Classify and Assess Shipments: Altana’s platform identifies the contents of shipping containers, classifies the goods, and provides compliance ratings for customs and security purposes.
- Enhance Visibility: Altana helps organizations gain detailed insights into the networks of suppliers and receivers involved in global trade, which is critical for compliance with laws like the Uyghur Forced Labor Prevention Act.
- Enable ‘Shared Intelligence’: The platform allows participants in the supply chain to share insights while protecting their proprietary data, ensuring privacy and security.
As enterprises seek to improve supply chain visibility and navigate complex global logistics, AI solutions like Altana’s are becoming indispensable. For businesses operating in highly regulated sectors, Altana’s ability to provide real-time, AI-driven insights into supply chain networks, without pooling sensitive data, is a game-changer. It offers a scalable solution to one of the most pressing challenges faced by companies—managing global operations in an increasingly interconnected and regulated world.
Altana’s AI systems have already garnered attention from organizations like UPS and several government agencies. Their use of AI to power a federated system of insights is a powerful example of how AI can fundamentally reshape the way companies manage global supply chains.
Traditional AI Limitations in Healthcare Workflows
Traditional AI models often fall short in addressing the highly regulated and fragmented workflows in healthcare, where integrating AI into everyday operations requires seamless data flow between various systems, such as Electronic Health Records (EHR) and practice management tools, while ensuring compliance with strict privacy regulations like HIPAA.
A company that is tackling this problem is Notable, a Greylock-backed Series B company transforming patient engagement and healthcare workflows by integrating AI deeply into its platform. Unlike many AI tools that only automate individual tasks, Notable combines advanced AI-driven automation with deep integration into existing healthcare systems, helping providers automate workflows like appointment scheduling, bill payments, and patient follow-ups.
- Collaborative Environment for AI-Powered Healthcare Solutions: Notable creates an environment where healthcare providers, administrative staff, and AI tools work together to manage patient data and streamline workflows. The system adapts to the complexities of healthcare by automating repetitive tasks while keeping healthcare professionals in control, ensuring that human judgment guides clinical decision-making.
- Seamless Integration with Legacy Systems: Notable offers pre-built, customizable solutions that integrate directly with EHR systems like Epic, making it possible to incorporate AI insights without overhauling existing infrastructure. This integration happens behind the scenes, allowing healthcare providers to use AI-driven tools that enhance patient experiences and operational efficiency without requiring technical expertise.
- Automated AI Model Development and Maintenance: Through its AI-powered assistant and large language models (LLMs), Notable continuously updates its algorithms to meet evolving patient and provider needs. This adaptability ensures that AI-driven workflows remain responsive to real-time healthcare demands, enhancing the accuracy, efficiency, and personalization of patient care while maintaining compliance with healthcare regulations.
Challenges and Barriers to Entry
While the benefits of deeply integrated AI solutions are clear, there are several challenges on the way toward achieving this level of adoption:
- Bureaucracy: Implementing an AI solution requires gaining buy-in from multiple stakeholders—ranging from department heads to legal teams and IT administrators. However, many enterprises often lack internal expertise in AI. This knowledge gap makes it even more difficult to gain the necessary buy-in or quickly implement AI, as stakeholders may be hesitant to approve new technologies they don’t fully understand. some text
- Our recommendation: Understand the level of AI literacy an organization has, and tailor your approach to bridge any knowledge gaps. Start by offering educational workshops or seminars to familiarize stakeholders with AI concepts and their practical applications.
- Security & Compliance: Whether handling sensitive customer information or integrating with existing enterprise systems, AI solutions need to comply with various national and international regulations. Validating data inputs, managing how data is used and shared, and ensuring that all processes adhere to compliance requirements are critical steps. some text
- Our recommendation: Prioritize obtaining industry-standard compliance certifications like SOC 2 Type II, as enterprise customers often require them before partnering. Leverage compliance automation platforms such as Vanta or WorkOS to streamline the certification process, saving time and resources. This proactive approach ensures regulatory compliance and builds trust with potential clients by demonstrating your commitment to security.
- Technical Complexity: Integrating AI with diverse enterprise systems is technically demanding. It requires expertise not only in AI but also in the legacy systems—such as ERP, TMS, or POS platforms—that AI must interact with. This complexity often necessitates substantial investment in both time and resources.some text
- Our recommendation: Understand your customers' integration needs and dependencies, and thoughtfully prioritize which systems to integrate with early on, considering factors like API approval wait times and security requirements. Collaborate closely with their technical teams and consider using middleware solutions to simplify the integration process. By building a strategic roadmap that addresses urgent integrations while concurrently preparing for more complex ones, you can efficiently manage resources and ensure successful AI integration with diverse enterprise systems.
- Understanding Multimodal Inputs: Enterprises have unique processes and requirements, meaning AI solutions must be customized to fit their specific operational context. For example, in logistics, AI must be tailored to manage different types of deliveries, varying regulatory requirements, and geographical conditions. This customization ensures AI remains effective as the business evolves.some text
- Our Recommendation: Build a data moat by combining different data inputs—or modalities—to customize AI solutions for your enterprise's unique operational context. By integrating diverse data types like text, images, and sensor readings, you enhance the AI's ability to handle specific processes, regulatory requirements, and geographical conditions. This multimodal approach not only improves effectiveness but also creates a competitive advantage that's difficult for competitors to replicate.
Successfully addressing these barriers is what separates high-impact, enterprise-ready AI solutions from commoditized tools. The key to long-term value lies in creating AI that doesn’t just offer insights but is fully embedded into an organization’s core processes, making it indispensable for daily operations.
Long-Term Value and Competitive Advantage
While there are significant challenges, companies that succeed in building and overcoming these challenges will play a pivotal role in the long-term value and defensibility of enterprise AI applications. These AI solutions don’t just solve current problems; they create foundational systems that are hard to displace and continually evolve to meet the growing demands of businesses with the following advantages:
- Long-Term Value and Defensibility: Companies that overcome AI integration challenges create foundational, hard-to-displace systems that evolve with business needs.
- High Barrier to Entry: Deeply integrated AI solutions require significant investment in time, expertise, and resources, raising the entry barrier for competitors.
- Lock-In and High Switching Costs: Once embedded, AI systems become difficult and costly to replace due to the initial investment in integration, training, and fine-tuning.
- Continuous Improvement: AI systems evolve with ongoing data input, refining predictions and optimizing workflows, making them increasingly indispensable.
- Strategic Asset: The continuous improvement cycle turns AI into a core part of decision-making, reinforcing its role as a long-term strategic advantage.
Conclusion
The future of AI in enterprises lies not in surface-level tools, but in deeply integrated solutions that become the backbone of operations. As companies move beyond simple, commoditized AI applications, those who invest in building solutions that embed AI into core processes will reap long-term rewards.
These AI systems are not just tools but strategic assets—transforming businesses by automating decision-making, optimizing workflows, and driving continuous improvement. The complexity involved in these integrations creates a durable competitive moat, raising barriers for rivals and locking in enterprises with high switching costs. In a world where data is gold, the enterprises that successfully navigate the technical and operational challenges of AI adoption will be the ones to define the future of business.